One Step Ahead! Fuji Electric's Fundamental Technologies
We provide an overview of our latest fundamental technologies that enhance our product development capabilities.
Forecast Technology Utilizing an Artificial Neural Network
Clarifying the correlation between forecast results and meteorological data
An artificial neural network is a computational model resulting from the computerized reproduction of a mechanism of information processing in the human brain. It forecasts results that are output when new information is added to data it has already learned. For a power demand forecast, the neural network learns the correlation between past power demand and meteorological data such as the temperature, humidity, and weather. From this correlation, it calculates power demand and the weather forecast for the day of the demand forecast, and outputs the result (Figure1).
The combinations of temperature, humidity, and other factors involved in learning the correlation are complicated, which previously made it difficult to identify how the respective factors affected the forecast results. Under such circumstances, Fuji Electric has leveraged the strength of our in-house capability to build computational models for neural networks, becoming the first in the industry to achieve a technology to learn each factor (Figure2). For example, we are able to analyze the power demand change in kW for a temperature increase/decrease of 1°C. We have also developed a technology to automatically eliminate unusual data unnecessary for the forecast that is included in the data used for learning, thereby improving the accuracy of the forecast. These efforts improved the convenience of the power demand forecast system. In the future, we intend to expand the scope of application of this forecast technology to utilization for temperature prediction of hot metal※ in steel plants, for example.
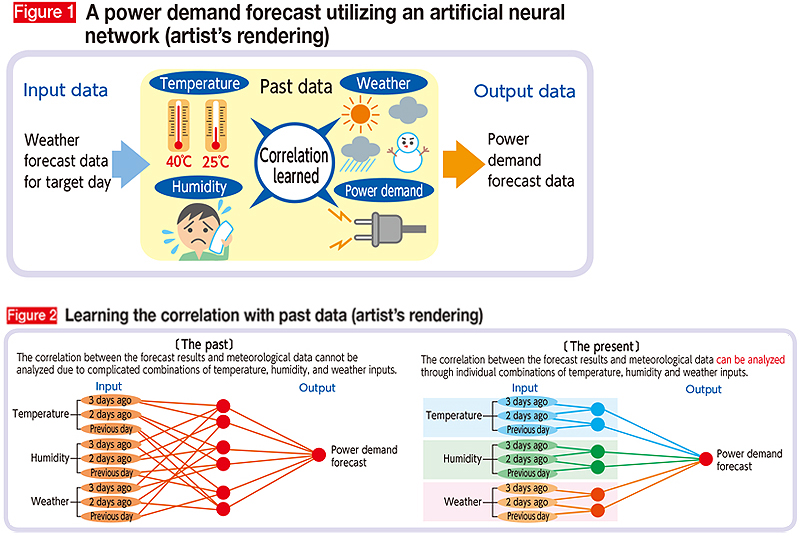
Contributing to a stable supply of power with a power demand forecast system
In electric power, it is necessary for the supply to match the demand at all times. Failure to maintain this supply-demand balance can affect the rotational speed of the generator at a power station. When demand exceeds supply, for example, the burden on the generator increases, causing the rotational speed to decrease. Such a change in the rotational speed can lead to metal fatigue of the rotating shaft of the generator, which can result in generator shutdown or even a massive blackout.
Power demand can vary greatly depending on the season, weather, and time of day. On the supply side, however, there is a trend to adopt more solar power and wind power generation in order to prevent global warming. These energy sources depend on natural conditions (the amount of sunlight and air volume and direction), making it difficult to quickly adjust the output. This poses a challenge to power producers in terms of careful power generation planning.
Against this backdrop, needs are increasing for a power demand forecast, which is essential to power generation planning. There is also a demand to make clear the correlation between the forecast results and meteorological data. FE is contributing to solving these issues with a power demand forecast system utilizing an artificial neural network.
-
*
-
Molten pig iron
Voices of developers
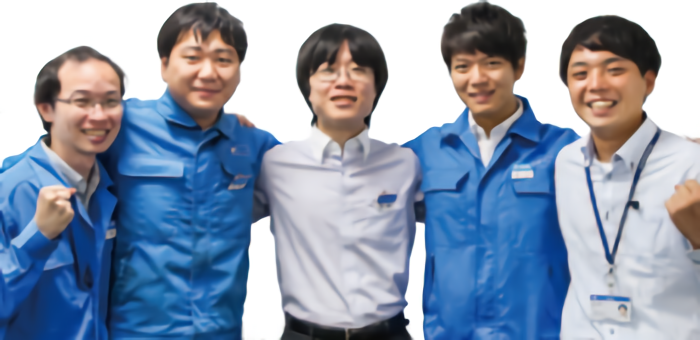
Digital Innovation Laboratory, Corporate R&D Headquarters
Our forecast system, which utilizes an artificial neural network, was mostly applied to major utility companies and other players in the power sector in the past.
Recently, however, new needs have arisen because of the manufacturing industry’s increasing interest in AI. In the future, we intend to develop a system that assists the manufacturing industry as well as the power sector with the improvement of product quality and labor saving to contribute to business expansion.
The article and the affiliations are at the time of coverage.